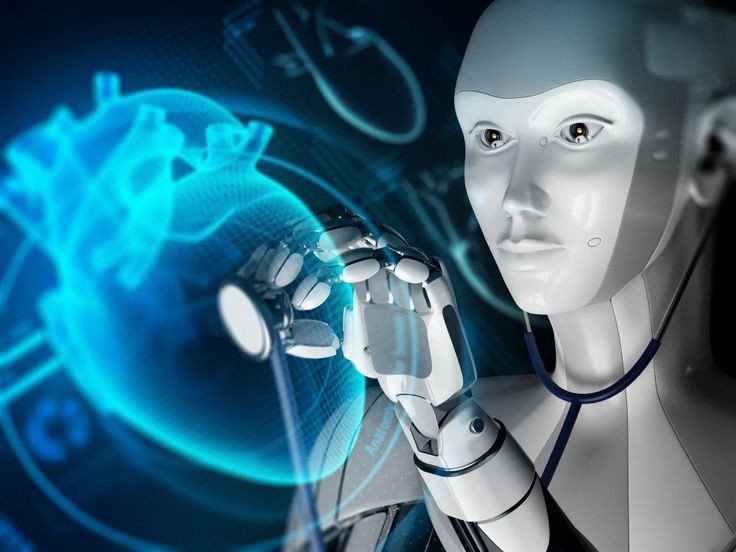
Data science has significantly transformed various industries, and healthcare is no exception. The integration of data science into healthcare has brought about numerous advancements, improving patient care, optimizing operations, and fostering innovation. This article explores the impact of data science on healthcare, highlighting its applications, benefits, challenges, and future trends.
Applications of Data Science in Healthcare
Predictive Analytics
Predictive analytics uses historical data to forecast future events. In healthcare, predictive models can:
– Predict disease outbreaks and epidemics.
– Forecast patient admission rates, helping hospitals manage resources.
– Identify patients at risk of developing chronic conditions, enabling early intervention.
Personalized Medicine
Personalized medicine tailors treatment plans to individual patients based on their genetic makeup, lifestyle, and other factors. Data science enables:
– Analysis of genetic data to identify the best treatment options.
– Development of personalized drug therapies.
– Prediction of how patients will respond to specific treatments.
Medical Imaging
Data science techniques, particularly deep learning, have revolutionized medical imaging. Applications include:
– Automated analysis of X-rays, MRIs, and CT scans to detect abnormalities.
– Enhanced image resolution and clarity.
– Early detection of diseases such as cancer through pattern recognition.
Electronic Health Records (EHRs)
EHRs store comprehensive patient data, including medical history, treatments, and test results. Data science helps:
– Improve the accuracy and completeness of EHRs.
– Identify patterns and trends in patient data for better clinical decision-making.
– Predict patient outcomes based on historical data.
Drug Discovery
The drug discovery process is traditionally time-consuming and expensive. Data science accelerates this process by:
– Analyzing biological data to identify potential drug targets.
– Predicting the efficacy and safety of new drugs.
– Optimizing clinical trials by identifying suitable participants and predicting outcomes.
Remote Monitoring and Telehealth
Remote monitoring and telehealth services have gained prominence, especially during the COVID-19 pandemic. Data science facilitates:
– Analysis of data from wearable devices to monitor patient health in real-time.
– Development of telehealth platforms that provide remote consultations and treatment.
– Identification of trends and patterns in patient data to improve remote care.
Benefits of Data Science in Healthcare
Improved Patient Outcomes
Data science enables early diagnosis, personalized treatment plans, and continuous monitoring, leading to better patient outcomes. Predictive analytics helps identify at-risk patients, allowing for timely intervention and preventive care.
Enhanced Operational Efficiency
Healthcare providers can optimize their operations through data-driven decision-making. Predictive models forecast patient admissions, resource requirements, and treatment outcomes, leading to more efficient resource allocation and reduced costs.
Accelerated Research and Innovation
Data science accelerates medical research by analyzing vast amounts of data quickly and accurately. This leads to faster drug discovery, improved clinical trials, and the development of innovative treatments and therapies.
Personalized Care
Personalized medicine, powered by data science, tailors treatments to individual patients based on their unique characteristics. This approach improves treatment efficacy, reduces adverse effects, and enhances patient satisfaction.
Better Disease Management
Data science provides insights into disease patterns and trends, enabling better disease management and prevention strategies. By identifying risk factors and predicting outbreaks, healthcare providers can implement targeted interventions.
Challenges of Data Science in Healthcare
Data Privacy and Security
Healthcare data is highly sensitive, and ensuring its privacy and security is paramount. Data breaches can have severe consequences, including identity theft and loss of patient trust. Healthcare organizations must comply with regulations such as HIPAA and GDPR to protect patient data.
Data Quality and Integration
The accuracy and reliability of data science models depend on high-quality data. However, healthcare data is often fragmented, inconsistent, and incomplete. Integrating data from various sources, such as EHRs, wearables, and clinical trials, poses significant challenges.
Ethical Considerations
Using data science in healthcare raises ethical questions, such as patient consent, data ownership, and algorithmic bias. Ensuring that data science practices are ethical and transparent is crucial for maintaining patient trust and equity in healthcare.
Technical and Infrastructure Challenges
Implementing data science solutions in healthcare requires robust technical infrastructure and skilled personnel. Many healthcare organizations face challenges in acquiring and maintaining the necessary technology and expertise.
Interpretability and Trust
Machine learning models, especially deep learning, can be complex and difficult to interpret. Healthcare professionals must trust these models to make critical decisions. Ensuring model transparency and explainability is essential for building trust among clinicians and patients.
Future Trends in Data Science in Healthcare
Integration of AI and Machine Learning
Artificial intelligence (AI) and machine learning (ML) will continue to play a significant role in healthcare. Future advancements include:
– More accurate predictive models for disease detection and treatment outcomes.
– Enhanced natural language processing (NLP) for analyzing unstructured data, such as clinical notes.
– Development of AI-driven diagnostics and decision support systems.
Genomics and Precision Medicine
The integration of genomics and data science will drive the future of precision medicine. Applications include:
– Comprehensive analysis of genetic data to develop targeted therapies.
– Identification of genetic markers for disease predisposition and drug response.
– Personalized treatment plans based on individual genetic profiles.
Wearable Technology and IoT
Wearable devices and the Internet of Things (IoT) will continue to transform healthcare by providing continuous monitoring and real-time data. Future trends include:
– Advanced analytics for processing data from wearables to predict health issues.
– Integration of IoT devices with EHRs for seamless data flow.
– Development of remote monitoring solutions for chronic disease management.
Telehealth and Remote Care
Telehealth and remote care will expand, driven by advancements in data science. Future trends include:
– Enhanced telehealth platforms with AI-driven diagnostic tools.
– Real-time analytics for monitoring patient health remotely.
– Integration of telehealth with other healthcare services for comprehensive care.
Ethical AI and Data Governance
As data science in healthcare advances, ensuring ethical AI practices and robust data governance will be crucial. Future trends include:
– Development of guidelines and frameworks for ethical AI in healthcare.
– Implementation of data governance policies to ensure data quality, security, and privacy.
– Promotion of transparency and accountability in AI and data science practices.
Conclusion
Data science has a profound impact on healthcare, driving improvements in patient outcomes, operational efficiency, and medical research. While challenges exist, ongoing advancements in technology and methodologies continue to enhance the capabilities and potential of data science in healthcare. By addressing privacy, security, and ethical concerns, healthcare organizations can harness the full power of data science to transform patient care and foster innovation. As the field evolves, staying abreast of emerging trends and best practices will be crucial for leveraging data science to achieve sustainable success in healthcare.