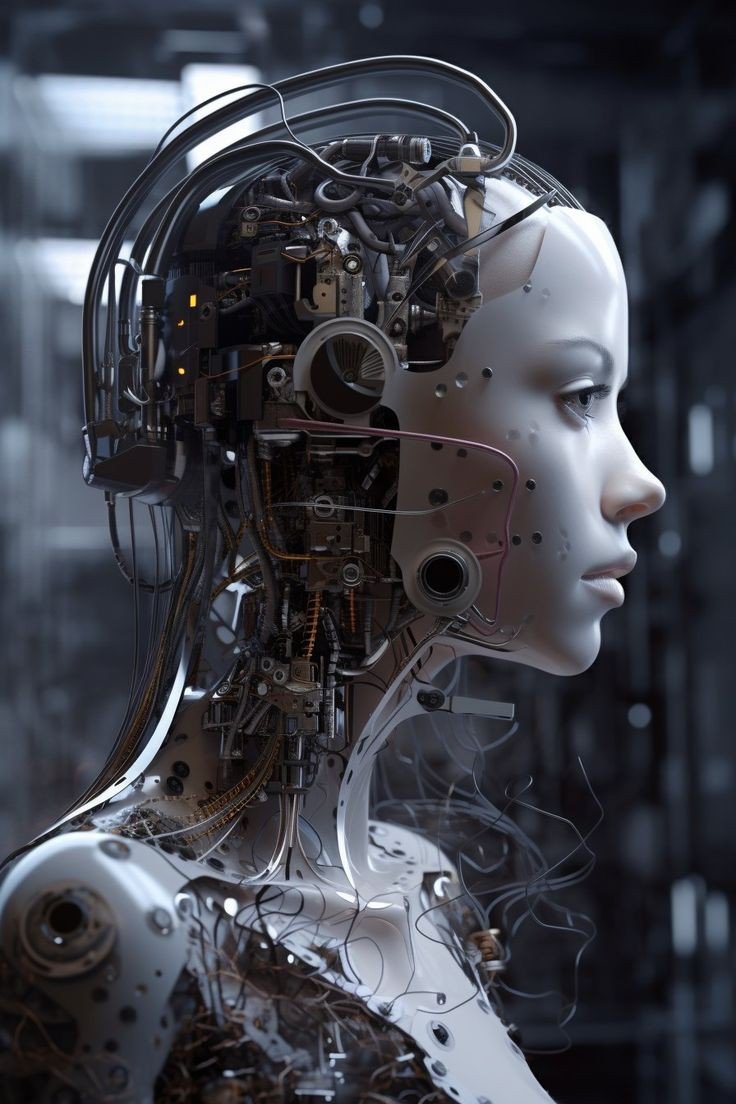
Machine learning (ML) has revolutionized numerous industries, offering unprecedented capabilities in data analysis, prediction, and automation. From healthcare to finance, machine learning algorithms drive innovation and efficiency. However, with great power comes great responsibility. The rise of machine learning has also brought about significant ethical concerns that must be addressed to ensure the technology benefits society without causing harm. This article explores the key ethical issues in machine learning, including bias, privacy, accountability, and transparency, and discusses ways to mitigate these concerns.
Understanding Ethical Concerns in Machine Learning
Ethical concerns in machine learning arise from the impact of algorithms on individuals and society. These concerns encompass a wide range of issues, including fairness, discrimination, privacy violations, and the potential for misuse. As machine learning systems become more integrated into daily life, understanding and addressing these ethical challenges is crucial.
Key Ethical Concerns
1. Bias and Discrimination: Machine learning models can inadvertently learn and perpetuate biases present in the training data, leading to discriminatory outcomes.
2. Privacy Violations: The use of personal data in machine learning raises significant privacy concerns, particularly when data is collected and used without consent.
3. Lack of Transparency: Many machine learning models, especially complex ones like deep neural networks, are often “black boxes,” making it difficult to understand how decisions are made.
4. Accountability: Determining who is responsible for the actions and decisions made by machine learning systems can be challenging.
5. Misuse of Technology: Machine learning can be used maliciously, such as for surveillance, deepfakes, or autonomous weapons, posing risks to security and society.
Bias and Discrimination in Machine Learning
Understanding Bias
Bias in machine learning refers to systematic errors that result in unfair outcomes. These biases can stem from various sources, including biased training data, algorithmic design, and deployment contexts. Common types of bias include:
1. Selection Bias: Occurs when the training data is not representative of the population the model will serve.
2. Measurement Bias: Arises from inaccuracies in the data collection process.
3. Label Bias: Happens when the labels in the training data reflect subjective judgments or stereotypes.
4. Algorithmic Bias: Results from the design and implementation of the algorithm itself.
Consequences of Bias
Bias in machine learning can have serious consequences, including discrimination in hiring, lending, and law enforcement. For example, biased facial recognition systems have been shown to have higher error rates for certain demographic groups, leading to potential wrongful arrests or surveillance.
Mitigating Bias
Addressing bias in machine learning requires a multi-faceted approach:
1. Diverse and Representative Data: Ensuring that training data is diverse and representative of all populations.
2. Bias Detection and Monitoring: Implementing tools and processes to detect and monitor bias throughout the model development lifecycle.
3. Fairness-Aware Algorithms: Developing and using algorithms designed to minimize bias and promote fairness.
4. Ethical Training: Educating data scientists and engineers about the importance of ethical considerations in their work.
Privacy Concerns in Machine Learning
Data Collection and Consent
Machine learning often relies on large amounts of personal data, raising concerns about how this data is collected and used. In many cases, individuals may not be aware that their data is being collected, or they may not have given explicit consent.
Data Security
Storing and processing large datasets pose significant security risks. Data breaches can lead to the exposure of sensitive information, causing harm to individuals and organizations.
Mitigating Privacy Risks
1. Data Minimization: Collecting only the data that is necessary for the intended purpose.
2. Anonymization and Encryption: Protecting data by anonymizing personal information and using encryption to secure data.
3. Transparent Policies: Clearly communicating data collection and usage policies to individuals and obtaining informed consent.
Transparency and Explainability
The Black Box Problem
Many machine learning models, particularly deep learning models, are often considered “black boxes” because their internal workings are not easily interpretable. This lack of transparency can make it difficult to understand how decisions are made and to identify potential biases.
Importance of Explainability
Explainability is crucial for building trust in machine learning systems. It allows stakeholders to understand the reasoning behind decisions, identify and address biases, and ensure that models are aligned with ethical standards.
Enhancing Transparency
1. Interpretable Models: Using models that are inherently more interpretable, such as decision trees or linear models, when possible.
2. Model Documentation: Documenting the development process, including data sources, model architecture, and evaluation metrics.
3. Explainability Tools: Developing and using tools that provide insights into model behavior and decision-making processes.
Accountability in Machine Learning
Responsibility and Liability
Determining who is responsible for the actions and decisions made by machine learning systems can be challenging. This includes issues of liability when machine learning systems cause harm or make incorrect decisions.
Ethical Frameworks
Developing ethical frameworks and guidelines can help clarify responsibilities and ensure that machine learning systems are used responsibly. These frameworks should address:
1. Accountability: Defining who is accountable for different aspects of the system, from data collection to deployment.
2. Governance: Establishing governance structures to oversee the ethical use of machine learning.
3. Ethical Audits: Conducting regular audits to ensure compliance with ethical standards.
Misuse of Machine Learning Technology
Surveillance and Privacy Invasion
Machine learning can be used for mass surveillance, posing significant privacy risks. Governments and organizations can use ML to monitor individuals’ activities, raising ethical and legal concerns.
Deepfakes and Misinformation
The ability to create realistic fake content, known as deepfakes, poses risks to privacy, security, and trust. Deepfakes can be used to spread misinformation, manipulate public opinion, and damage reputations.
Autonomous Weapons
The development of autonomous weapons using machine learning technology raises significant ethical and security concerns. These weapons could make independent decisions about life and death, leading to potential misuse and loss of human control.
Mitigating Misuse
1. Regulatory Measures: Implementing regulations to govern the use of machine learning in sensitive areas such as surveillance and autonomous weapons.
2. Ethical Guidelines: Developing and adhering to ethical guidelines that outline acceptable and unacceptable uses of machine learning technology.
3. Public Awareness: Raising awareness about the potential risks and misuse of machine learning technology.
Conclusion
Machine learning offers tremendous potential for innovation and progress, but it also brings significant ethical challenges that must be addressed. By understanding and mitigating bias, ensuring privacy, enhancing transparency, establishing accountability, and preventing misuse, we can harness the power of machine learning responsibly and ethically. As machine learning continues to evolve, ongoing dialogue, research, and collaboration will be essential to navigate the complex ethical landscape and ensure that this powerful technology serves the greater good.
ALSO READ: AI in Modern Healthcare: Role, Application, and More