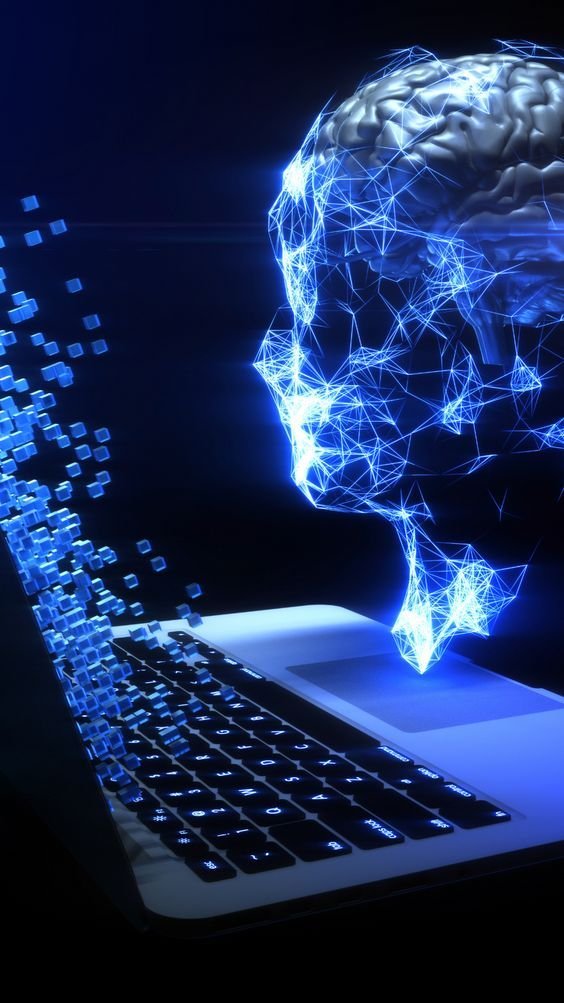
The field of sports analytics has seen significant advancements with the advent of data science. Data science techniques are being utilized to enhance athletic performance, inform strategic decisions, and improve fan engagement. This article explores the various ways data science is revolutionizing sports analytics, including key methodologies, applications, benefits, challenges, and future trends.
Key Methodologies in Sports Analytics
Data Collection
Data collection is the foundational step in sports analytics. It involves gathering data from various sources such as sensors, video feeds, and manual logs. Common sources of sports data include:
– Wearable devices: Track player movements, heart rate, and other physiological metrics.
– Video analysis: Cameras capture in-game actions and player movements.
– Official records: Game statistics, player performance data, and historical records.
– Social media: Fan interactions and sentiment.
Data Processing and Cleaning
The collected data often comes in raw, unstructured formats. Data processing and cleaning involve transforming this raw data into a structured format suitable for analysis. This step includes:
– Data normalization: Ensuring consistency in data formats.
– Handling missing values: Imputing or removing incomplete data entries.
– Data integration: Combining data from multiple sources.
Exploratory Data Analysis (EDA)
EDA is used to understand the underlying patterns and characteristics of the data. It involves:
– Descriptive statistics: Summarizing data using mean, median, mode, standard deviation, etc.
– Data visualization: Creating charts and graphs to visualize trends and patterns.
– Correlation analysis: Identifying relationships between different variables.
Predictive Modeling
Predictive modeling involves using statistical and machine learning techniques to forecast future outcomes based on historical data. Common predictive models in sports analytics include:
– Regression analysis: Predicting continuous outcomes like player performance scores.
– Classification algorithms: Categorizing outcomes such as win/loss or player positions.
– Time series analysis: Forecasting future events based on past trends, such as game outcomes.
Machine Learning and AI
Advanced machine learning and AI techniques are employed to gain deeper insights and automate decision-making processes. Key techniques include:
– Neural networks: Used for complex pattern recognition and predictive tasks.
– Clustering: Grouping similar data points to identify patterns, such as player types or team strategies.
– Natural Language Processing (NLP): Analyzing textual data from social media or player interviews.
Applications of Data Science in Sports Analytics
Player Performance Analysis
Data science helps in assessing and improving player performance by analyzing various metrics collected from games and training sessions. Applications include:
– Injury prediction and prevention: Using historical data to identify risk factors and prevent injuries.
– Skill development: Analyzing performance data to tailor training programs for individual players.
– Fitness monitoring: Tracking physiological metrics to optimize player fitness and recovery.
Team Strategy and Tactics
Coaches and analysts use data science to devise game strategies and tactics. Applications include:
– Opponent analysis: Studying opponents’ strengths and weaknesses to develop effective game plans.
– In-game decision-making: Real-time data analysis to make tactical decisions during games.
– Formation optimization: Analyzing data to determine the most effective team formations and lineups.
Fan Engagement and Experience
Data science enhances fan engagement by providing personalized experiences and insights. Applications include:
– Sentiment analysis: Analyzing social media data to gauge fan sentiment and feedback.
– Personalized content: Delivering tailored content to fans based on their preferences and behaviors.
– Ticket pricing: Using dynamic pricing models to optimize ticket sales and revenue.
Business and Operations
Sports organizations use data science to streamline their operations and improve financial performance. Applications include:
– Revenue optimization: Analyzing data to maximize revenue from ticket sales, merchandise, and sponsorships.
– Resource allocation: Optimizing the allocation of resources such as training facilities and equipment.
– Marketing strategies: Developing data-driven marketing campaigns to attract and retain fans.
Benefits of Data Science in Sports Analytics
Improved Performance and Decision-Making
Data-driven insights enable coaches, players, and teams to make informed decisions, leading to improved performance and outcomes. Predictive analytics helps in anticipating future events and preparing accordingly.
Enhanced Fan Engagement
Personalized content and experiences keep fans engaged and loyal to their favorite teams and players. Analyzing fan sentiment helps organizations understand and respond to fan needs and preferences.
Optimized Resource Utilization
Data science helps in optimizing the use of resources, whether it’s player training schedules, marketing budgets, or stadium operations. This leads to cost savings and increased efficiency.
Competitive Advantage
Teams that leverage data science effectively gain a competitive edge over their rivals. By uncovering hidden patterns and insights, they can develop innovative strategies and tactics.
Risk Mitigation
Predictive analytics helps in identifying and mitigating risks, such as player injuries or financial losses. This proactive approach ensures better preparedness and resilience.
Challenges in Sports Analytics
Data Quality and Availability
The accuracy and reliability of analytics depend on the quality and availability of data. Incomplete or inconsistent data can lead to incorrect insights and decisions.
Integrating Diverse Data Sources
Combining data from multiple sources, such as wearables, video feeds, and social media, can be challenging. Ensuring data compatibility and integration is crucial for comprehensive analysis.
Privacy and Ethical Concerns
Collecting and analyzing personal data from players and fans raises privacy and ethical concerns. Organizations must ensure compliance with data protection regulations and ethical standards.
Skill Gaps
Implementing advanced data science techniques requires specialized skills in data analysis, machine learning, and domain knowledge. Bridging these skill gaps is essential for successful analytics projects.
Keeping Up with Technological Advances
The field of data science is rapidly evolving, with new tools and techniques emerging regularly. Staying updated with the latest advancements is crucial for maintaining a competitive edge.
Future Trends in Sports Analytics
Real-Time Analytics
The demand for real-time analytics is growing, enabling teams to make immediate decisions based on live data. This includes in-game tactics, injury management, and fan engagement.
Advanced Wearable Technology
Wearable devices are becoming more sophisticated, providing detailed physiological and biomechanical data. This enables deeper insights into player performance and health.
Integration with Augmented and Virtual Reality (AR/VR)
AR and VR technologies are being integrated with sports analytics to provide immersive training experiences and enhance fan engagement. This includes virtual coaching and interactive fan experiences.
AI and Machine Learning Advancements
Continued advancements in AI and machine learning will enable more accurate predictions, automated decision-making, and deeper insights. This includes the use of deep learning and reinforcement learning techniques.
Blockchain for Data Security
Blockchain technology is being explored for securing and authenticating sports data. This ensures data integrity and trustworthiness, particularly in areas like player contracts and anti-doping measures.
Conclusion
Data science is transforming the landscape of sports analytics, providing valuable insights that enhance player performance, team strategies, fan engagement, and operational efficiency. While challenges exist, the benefits of leveraging data science in sports are immense. As technology continues to advance, the field of sports analytics will continue to evolve, offering new opportunities for innovation and competitive advantage. By staying updated with emerging trends and best practices, sports organizations can harness the full potential of data science to achieve their goals and drive success.
ALSO READ: Ethical Concerns in Machine Learning