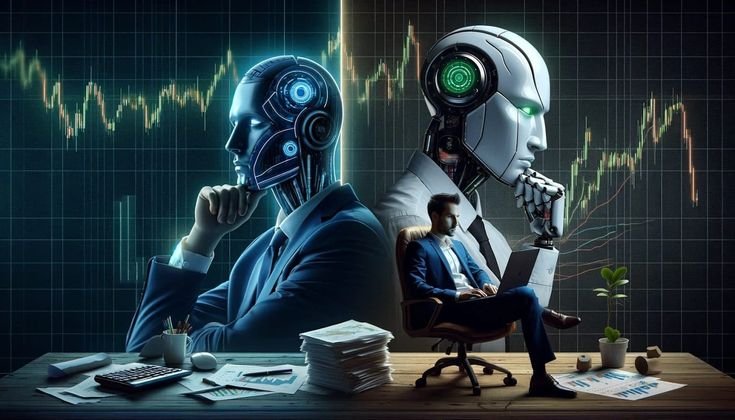
PathOrchestra: Revolutionizing AI-Assisted Disease Diagnosis in China
In a groundbreaking development, a Chinese research team has created the nation’s first versatile artificial intelligence (AI) model proficient in analyzing a wide array of pathological images. This model, known as PathOrchestra, signifies a transformative shift in AI-assisted disease diagnosis, moving from single-cancer-specific models to one capable of addressing multiple cancers. Researchers from Air Force Medical University (AFMU), Tsinghua University, and SenseTime collaborated on this project, utilizing China’s largest domestic dataset of digital pathology images. This article delves into the details of PathOrchestra, its development, capabilities, and the potential impact on medical diagnostics.
The Genesis of PathOrchestra
Collaborating Institutions
The creation of PathOrchestra was a joint effort by three major institutions: the Air Force Medical University (AFMU), Tsinghua University, and SenseTime. Each of these institutions brought unique expertise to the project:
1. Air Force Medical University (AFMU): Known for its extensive medical research, AFMU provided the clinical insight and pathological expertise necessary to guide the AI’s learning process.
2. Tsinghua University: One of China’s premier research universities, Tsinghua contributed advanced AI and machine learning capabilities.
3. SenseTime: As a leading AI company, SenseTime offered cutting-edge technology and computational resources.
The Dataset
PathOrchestra was trained using the largest domestic dataset of digital pathology images in China, comprising nearly 300,000 whole-slide images. This dataset is a remarkable 300 terabytes in size, providing a vast amount of data for the AI to learn from. The diversity and volume of this dataset are critical in training a model capable of analyzing different types of pathological images across multiple organs.
The Technology Behind PathOrchestra
Self-Supervised Learning
One of the key technologies employed in PathOrchestra is self-supervised learning. Unlike traditional supervised learning, which requires labeled data, self-supervised learning allows the model to learn patterns and features from unlabeled data. This approach is particularly advantageous when dealing with large datasets like those used for PathOrchestra.
Cross-Learning
The model employs a technique known as “cross-learning,” which enables it to analyze over 20 different organs. By learning from the diverse range of pathological images, PathOrchestra can perform multiple clinical tasks, including:
– Pan-Cancer Classification: Identifying various types of cancers.
– Lesion Identification and Detection: Spotting and diagnosing lesions in different organs.
– Multi-Cancer Subtype Differentiation: Distinguishing between subtypes of cancers.
– Biomarker Assessment: Evaluating specific biological markers that can indicate the presence of disease.
Achievements and Accuracy
PathOrchestra has achieved an accuracy rate exceeding 95 percent in nearly 50 clinical tasks, including:
– Lymphoma Subtype Diagnosis: Differentiating between various subtypes of lymphoma, a type of blood cancer.
– Bladder Cancer Screening: Identifying signs of bladder cancer in pathological images.
These high accuracy rates are a testament to the model’s robustness and its potential to significantly aid pathologists in diagnosing diseases more quickly and accurately.
Overcoming Challenges in Pathological Image Analysis
Complexity of Pathological Images
Pathological images are highly complex and varied, making them a challenging target for AI analysis. The diversity in tissue types, staining techniques, and the presence of various artifacts can complicate image processing. According to Professor Wang Zhe from the Basic Medical Science Academy under the AFMU, this complexity has earned pathological image analysis the title of the “jewel in the crown” in image processing.
Addressing the Challenges
PathOrchestra addresses these challenges through its advanced learning techniques and the extensive dataset it was trained on. By cross-learning from a wide range of images, the model can recognize and adapt to the variations in pathological images, improving its diagnostic accuracy.
Implications for the Medical Field
Reducing Pathologists’ Workload
One of the significant benefits of PathOrchestra is its potential to reduce the workload of pathologists. The model can quickly and accurately analyze large volumes of images, allowing pathologists to focus on more complex cases and reducing the time needed for routine analyses.
Increasing Efficiency in Medical Image Review
By automating the initial review of medical images, PathOrchestra can significantly increase the efficiency of the diagnostic process. This improvement is particularly valuable in settings with limited medical personnel or high patient volumes, where quick and accurate diagnoses are essential.
Enhancing Diagnostic Accuracy
The high accuracy rate of PathOrchestra means that it can serve as a reliable tool for pathologists, reducing the likelihood of diagnostic errors. This reliability can lead to better patient outcomes, as diseases can be identified and treated more effectively.
PathOrchestra in the Context of Global AI Development
China’s Growing AI Landscape
PathOrchestra represents just one example of the rapid growth of AI technology in China. According to a recent white paper by the China Academy of Information and Communications Technology, China accounts for 36 percent of the more than 1,300 AI large language models (LLMs) globally, the second-largest proportion after the United States. This growth is indicative of China’s commitment to advancing AI technology across various sectors.
Comparison with Other AI Models
While many AI models are designed for specific tasks or types of cancer, PathOrchestra’s versatility sets it apart. Its ability to analyze multiple types of pathological images and perform various clinical tasks makes it a pioneering model in the field of AI-assisted disease diagnosis.
Future Prospects
Integration into Clinical Practice
The next step for PathOrchestra is its integration into clinical practice. This process will involve further validation of the model in real-world settings and training medical personnel to use the technology effectively. Once integrated, PathOrchestra has the potential to revolutionize the way diseases are diagnosed and managed.
Expansion to Other Medical Fields
The principles and techniques used in PathOrchestra could be applied to other areas of medical diagnostics. For instance, similar models could be developed to analyze radiology images, genetic data, or other types of medical information, broadening the impact of AI in healthcare.
Continuous Improvement
As with all AI models, continuous improvement is essential. PathOrchestra will need to be updated and refined as new data becomes available and as medical knowledge advances. This ongoing development will ensure that the model remains at the forefront of medical diagnostics.
Conclusion
PathOrchestra stands as a significant advancement in the field of AI-assisted disease diagnosis. Its development by a collaborative team from AFMU, Tsinghua University, and SenseTime, leveraging a massive dataset and cutting-edge learning techniques, marks a new era in medical image analysis. With its high accuracy and versatile capabilities, PathOrchestra has the potential to greatly reduce the workload of pathologists, increase diagnostic efficiency, and improve patient outcomes. As part of China’s rapidly growing AI landscape, PathOrchestra exemplifies the country’s commitment to advancing technology and its application in healthcare. The future prospects for this technology are vast, promising further innovations and improvements in medical diagnostics and beyond.
ALSO READ: China’s Push for Global AI Standards Gains UN Support