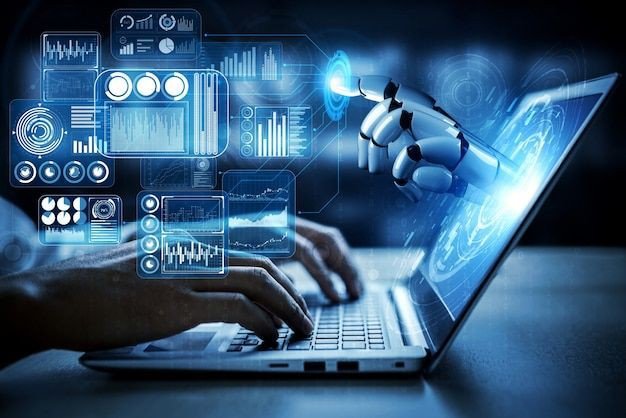
The process of drug discovery and development has traditionally been lengthy, costly, and complex, often taking years and billions of dollars to bring a new drug to market. With the advent of artificial intelligence (AI), the pharmaceutical industry is undergoing a transformative change. AI technologies, including machine learning, natural language processing, and deep learning, are revolutionizing how drugs are discovered and developed. This article explores the role of AI in drug discovery and development, highlighting its potential benefits, applications, and the challenges it faces.
The Role of AI in Drug Discovery and Development
AI’s primary role in drug discovery and development is to accelerate the process by which new therapeutic compounds are identified and optimized. By analyzing vast amounts of biological data, AI can identify potential drug candidates more quickly and accurately than traditional methods. The applications of AI in this field span several key areas, including target identification, drug design, prediction of drug efficacy and safety, and optimization of clinical trials.
Target Identification
One of the first steps in drug discovery is identifying biological targets—typically proteins or genes associated with a disease. AI can analyze genomic, proteomic, and other biological data to identify these targets. Machine learning algorithms can detect patterns and relationships within complex datasets that might not be apparent to human researchers. For example, AI can identify genetic mutations associated with specific diseases, helping researchers focus on relevant biological targets for drug development.
Drug Design and Molecular Simulation
AI has significantly impacted the design and simulation of new drug molecules. Traditional drug design involves the iterative process of creating and testing chemical compounds, which can be time-consuming and costly. AI algorithms, particularly deep learning models, can predict the chemical properties of compounds and their interactions with biological targets. This allows researchers to virtually screen thousands of compounds, identifying those most likely to bind effectively to the target.
Generative AI models, such as generative adversarial networks (GANs) and variational autoencoders (VAEs), can generate novel molecular structures with desired properties. These models can optimize the chemical structure of potential drugs to improve their efficacy, reduce side effects, and increase bioavailability. This capability accelerates the drug design process and opens new avenues for discovering previously unexplored chemical space.
Prediction of Drug Efficacy and Safety
Assessing a drug’s efficacy and safety is a critical aspect of drug development. AI can predict how potential drug candidates will behave in the human body, including their pharmacokinetics (absorption, distribution, metabolism, and excretion) and pharmacodynamics (mechanism of action and effect on the body). Machine learning models can analyze preclinical data, such as animal studies and in vitro experiments, to predict a compound’s efficacy in humans.
AI can also identify potential side effects and toxicities by analyzing chemical structure data and historical records of known drugs. This predictive capability helps in the early identification of safety concerns, reducing the likelihood of costly late-stage failures in clinical trials. By predicting drug efficacy and safety more accurately, AI can improve the success rate of drug development programs.
Optimization of Clinical Trials
Clinical trials are a critical and resource-intensive phase of drug development. AI can optimize various aspects of clinical trials, from patient recruitment to study design and data analysis. For example, AI algorithms can analyze patient data to identify individuals who are most likely to benefit from a new treatment, improving the efficiency of patient recruitment. This targeted approach can reduce the time and cost of trials.
AI can also help design adaptive clinical trials, where the trial protocol can be modified based on interim results. This flexibility allows for more efficient use of resources and can accelerate the approval of effective treatments. Additionally, AI-driven analytics can monitor trial data in real-time, identifying trends and anomalies that may require attention, thus ensuring the trial’s integrity and safety.
Repurposing Existing Drugs
Drug repurposing involves finding new therapeutic uses for existing drugs. This approach can significantly reduce the time and cost associated with bringing a new drug to market, as repurposed drugs have already undergone extensive testing for safety. AI can analyze large datasets, including clinical trial data, electronic health records, and scientific literature, to identify potential new uses for approved drugs. By uncovering hidden connections between drugs and diseases, AI can lead to the discovery of new treatments for conditions that currently lack effective therapies.
Benefits of AI in Drug Discovery and Development
The integration of AI into drug discovery and development offers several key benefits:
Accelerated Drug Discovery
AI can significantly speed up the drug discovery process by automating complex tasks and analyzing large datasets. What used to take years can now be accomplished in months, enabling faster identification of promising drug candidates.
Cost Reduction
The traditional drug discovery process is expensive, with high failure rates contributing to the overall cost. AI can reduce these costs by improving the accuracy of predictions regarding drug efficacy and safety, thereby decreasing the likelihood of costly late-stage failures.
Improved Precision and Personalization
AI enables the development of precision medicine by analyzing patient data to identify the most effective treatments for specific individuals. This personalized approach can improve treatment outcomes and reduce adverse effects.
Discovery of Novel Compounds
AI can explore vast chemical spaces and generate novel compounds with desired properties. This capability can lead to the discovery of entirely new classes of drugs, expanding the range of potential treatments for various diseases.
Challenges and Limitations
Despite its promise, the use of AI in drug discovery and development is not without challenges:
Data Quality and Accessibility
AI models rely on high-quality data for training and validation. In the pharmaceutical industry, data can be fragmented, incomplete, or inconsistent, making it difficult to obtain reliable results. Additionally, access to proprietary datasets can be restricted, limiting the amount of data available for analysis.
Interpretability and Transparency
AI algorithms, especially deep learning models, can be complex and difficult to interpret. This “black box” nature can make it challenging to understand how a model arrived at a particular prediction, which is critical for gaining regulatory approval and trust from healthcare professionals.
Regulatory and Ethical Considerations
The use of AI in drug development raises regulatory and ethical questions, particularly regarding data privacy and the transparency of AI-driven decisions. Regulatory agencies are still developing guidelines for evaluating AI-based tools, and there is a need for standardized frameworks to ensure compliance and patient safety.
Integration with Existing Workflows
Integrating AI technologies into existing drug discovery and development workflows can be challenging. It requires collaboration between AI experts, pharmaceutical researchers, and clinicians, as well as changes to established processes and infrastructure.
The Future of AI in Drug Discovery and Development
The future of AI in drug discovery and development is bright, with continued advancements in AI technologies and increasing investment in this area. Several trends are likely to shape the future of AI-driven drug discovery:
Expansion of AI Applications
As AI technologies continue to evolve, their applications in drug discovery and development will expand. For example, AI could play a more significant role in understanding disease mechanisms at the molecular level, identifying new drug targets, and predicting patient responses to treatment.
Collaboration and Open Innovation
Collaboration between pharmaceutical companies, academic institutions, and technology firms will be crucial for advancing AI-driven drug discovery. Open innovation models, where data and resources are shared among stakeholders, can accelerate research and development efforts.
Integration with Emerging Technologies
AI will increasingly be integrated with other emerging technologies, such as genomics, CRISPR gene editing, and personalized medicine. These synergies will enhance the ability to develop targeted therapies and address complex diseases.
Regulatory Framework Development
Regulatory agencies will continue to develop frameworks for evaluating and approving AI-driven drug discovery tools. These frameworks will need to address issues such as data quality, model validation, and transparency to ensure patient safety and public trust.
Conclusion
AI is poised to revolutionize drug discovery and development, offering the potential to accelerate the identification of new drugs, reduce costs, and improve treatment outcomes. By leveraging AI’s capabilities in data analysis, prediction, and optimization, the pharmaceutical industry can overcome many of the challenges associated with traditional drug development processes.
However, realizing the full potential of AI in this field requires addressing challenges related to data quality, interpretability, regulatory compliance, and integration with existing workflows. As technology advances and the industry adapts, AI-driven drug discovery and development will likely become a cornerstone of pharmaceutical research, leading to new and more effective therapies for patients worldwide.
ALSO READ: The Role of Blockchain in Supply Chain Management