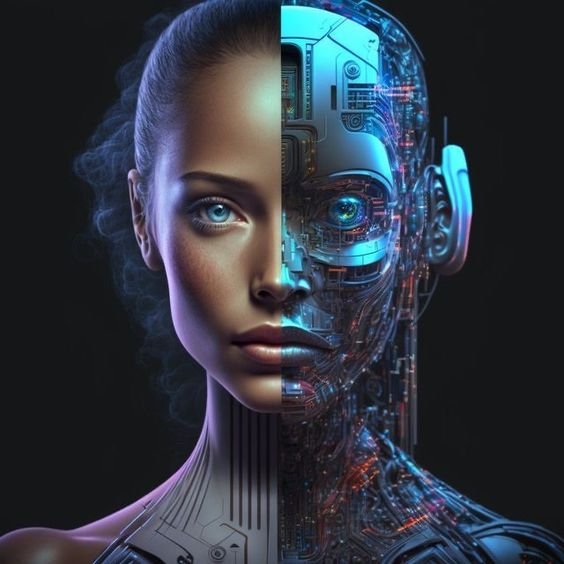
Industry 4.0, also known as the Fourth Industrial Revolution, is characterized by the integration of digital technologies into manufacturing and production processes. One of the most transformative aspects of Industry 4.0 is the use of artificial intelligence (AI) in predictive maintenance. Predictive maintenance uses data analysis tools and techniques to detect anomalies and predict equipment failures before they occur, thereby minimizing downtime and reducing maintenance costs. This article explores the role of AI in predictive maintenance within the context of Industry 4.0, its benefits, implementation strategies, and future prospects.
The Concept of Predictive Maintenance
Predictive maintenance is a proactive approach that uses data from various sensors and systems to monitor the condition of equipment and predict potential failures. Unlike reactive maintenance, which occurs after a failure, or preventive maintenance, which follows a scheduled plan, predictive maintenance aims to predict when equipment failure might occur and to perform maintenance just in time to prevent it. AI plays a crucial role in enhancing the accuracy and effectiveness of predictive maintenance by analyzing vast amounts of data and identifying patterns that human analysts might miss.
How AI Enhances Predictive Maintenance
– Data Collection and Processing: AI systems can collect and process data from multiple sources, such as sensors, machine logs, and historical maintenance records. This data includes temperature, vibration, pressure, and other parameters that indicate the health of machinery.
– Pattern Recognition: AI algorithms, particularly those based on machine learning, can identify patterns and correlations in the data that are indicative of potential failures. These algorithms can learn from past data to improve their predictive accuracy over time.
– Anomaly Detection: AI can detect anomalies or deviations from normal operating conditions. By identifying these anomalies early, maintenance teams can address potential issues before they lead to equipment failure.
– Predictive Analytics: Advanced AI models can predict the remaining useful life of equipment and estimate the time to failure. This allows maintenance to be scheduled at the optimal time, reducing unnecessary maintenance activities and preventing unexpected breakdowns.
– Decision Support: AI systems can provide maintenance teams with actionable insights and recommendations. These insights help prioritize maintenance tasks and allocate resources more effectively.
Benefits of AI-Powered Predictive Maintenance
– Reduced Downtime: By predicting and preventing equipment failures, AI-powered predictive maintenance significantly reduces unplanned downtime. This leads to increased productivity and efficiency in manufacturing processes.
– Cost Savings: Predictive maintenance helps avoid costly repairs and replacements by addressing issues before they escalate. It also reduces the need for excessive preventive maintenance, which can be resource-intensive.
– Extended Equipment Lifespan: Regular monitoring and timely maintenance can extend the lifespan of equipment. AI can help ensure that machinery operates within optimal parameters, reducing wear and tear.
– Improved Safety: Predictive maintenance enhances workplace safety by preventing catastrophic equipment failures that could lead to accidents or injuries.
– Resource Optimization: AI-driven predictive maintenance allows for better allocation of maintenance resources, including labor, spare parts, and time. This optimization leads to more efficient operations and lower overall maintenance costs.
Implementing AI-Powered Predictive Maintenance
Implementing AI-powered predictive maintenance requires a strategic approach that encompasses several key steps:
1. Assessment and Planning: The first step is to assess the current maintenance practices and identify the potential benefits of predictive maintenance. This involves understanding the critical equipment, failure modes, and data availability.
2. Data Collection and Integration: Effective predictive maintenance relies on high-quality data. Organizations need to deploy sensors and data collection systems to gather relevant information from equipment. This data should be integrated into a centralized system for analysis.
3. AI Model Development: Developing AI models for predictive maintenance involves selecting the appropriate machine learning algorithms and training them on historical data. The models should be capable of identifying patterns and predicting failures accurately.
4. Deployment and Monitoring: Once the AI models are developed, they need to be deployed in the production environment. Continuous monitoring and evaluation are essential to ensure the models perform as expected and to make adjustments as needed.
5. Integration with Maintenance Systems: AI predictive maintenance systems should be integrated with existing maintenance management systems. This integration allows for seamless scheduling of maintenance tasks and better coordination between maintenance teams.
6. Training and Change Management: Implementing predictive maintenance requires a cultural shift within the organization. Maintenance teams need to be trained on new tools and technologies, and there should be a focus on change management to ensure smooth adoption.
Challenges and Solutions
While AI-powered predictive maintenance offers numerous benefits, there are also challenges to consider:
– Data Quality and Availability: The effectiveness of AI models depends on the quality and availability of data. Organizations must invest in reliable data collection systems and ensure data is accurate and complete.
– Integration Complexity: Integrating AI systems with existing infrastructure can be complex. Organizations need to ensure compatibility and interoperability between different systems.
– Model Accuracy and Reliability: AI models need to be accurate and reliable to provide actionable insights. Continuous monitoring and retraining of models are necessary to maintain their effectiveness.
– Cost and ROI: Implementing AI-powered predictive maintenance requires significant investment. Organizations need to carefully evaluate the return on investment and ensure that the benefits outweigh the costs.
– Cybersecurity: The increased connectivity of AI systems raises cybersecurity concerns. Organizations must implement robust security measures to protect sensitive data and prevent cyberattacks.
Case Studies
– General Electric (GE): GE has implemented AI-powered predictive maintenance across its industrial operations. By using AI to monitor equipment health and predict failures, GE has reduced downtime and maintenance costs, leading to significant improvements in operational efficiency.
– Siemens: Siemens uses AI and machine learning to enhance predictive maintenance for its manufacturing and energy operations. The company’s MindSphere platform collects and analyzes data from connected devices, enabling predictive maintenance and optimizing performance.
– BMW: BMW leverages AI for predictive maintenance in its manufacturing plants. AI algorithms analyze data from machinery and equipment to predict failures and schedule maintenance, ensuring smooth production processes and reducing downtime.
Future Prospects
The future of AI in predictive maintenance is promising, with several advancements on the horizon:
– Advanced Analytics and Machine Learning: Ongoing advancements in machine learning and analytics will improve the accuracy and reliability of predictive maintenance models. These technologies will enable more precise predictions and early detection of potential failures.
– Integration with IoT: The integration of AI with the Internet of Things (IoT) will enhance predictive maintenance capabilities. IoT devices can provide real-time data, enabling continuous monitoring and immediate response to anomalies.
– Edge Computing: Edge computing will allow data processing to occur closer to the source, reducing latency and improving the speed of predictive maintenance systems. This will enable real-time analysis and decision-making.
– Enhanced Human-Machine Collaboration: Future predictive maintenance systems will facilitate better collaboration between humans and machines. AI will provide maintenance teams with more intuitive and actionable insights, enhancing their decision-making capabilities.
– Sustainability and Environmental Impact: AI-powered predictive maintenance can contribute to sustainability by optimizing equipment performance and reducing energy consumption. This will lead to lower environmental impact and support green manufacturing initiatives.
Conclusion
AI-powered predictive maintenance is a cornerstone of Industry 4.0, offering significant benefits in terms of reduced downtime, cost savings, extended equipment lifespan, and improved safety. While there are challenges to overcome, such as data quality and integration complexity, the potential advantages make it a worthwhile investment for forward-thinking organizations. As AI technology continues to evolve, predictive maintenance will become even more accurate and effective, driving further improvements in industrial efficiency and sustainability. By embracing AI in predictive maintenance, industries can stay ahead of the curve and fully realize the potential of the Fourth Industrial Revolution.
ALSO READ: Sneak Peek into Strawberry: New AI Model by OpenAI